Search Engine Result Page (SERP) analysis is an important aspect of digital marketing and SEO. It helps online businesses to understand how their online properties are ranking for specific keywords and provides insights into the overall performance of their websites. With the rise of machine learning, online businesses can now incorporate this amazing technology into their SERP analysis to gain more accurate and valuable insights. So let’s explore the integration of machine learning into SERP analysis and the numerous advantages it offers.
Keyword Ranking Analysis using machine learning
Machine learning algorithms can be used to analyse keyword rankings and provide a more accurate representation of a website’s online visibility. By analysing large amounts of data that can be collected through various SEO tools like Google Search Console or SERanking, machine learning algorithms can identify patterns and trends in the ranking of specific keywords. This information can then be used by digital marketers to optimise their website’s content and improve its online visibility in the SERPs.
A frequently used algorithm for keyword ranking analysis is regression analysis. This type of machine learning algorithm can be used to identify patterns in the ranking of specific keywords and provide insights into factors that are impacting their ranking.
Another algorithm that can be used for keyword ranking analysis is decision trees. This type of algorithm can be used to identify the most important factors affecting keyword rankings so SEOs can prioritise their efforts.
Machine learning for SEO competitor research
Machine learning algorithms can also be used to analyse the performance of online competitors. By analysing large amounts of data, machine learning algorithms can identify the tactics strategies used by online competitors to rank higher in the SERPs. This information can then be used to improve a website’s own ranking and gain a competitive advantage.
For competitor analysis in the Search Engine Result Pages, a good machine learning algorithm would be one that can effectively analyse keyword rankings, backlink analysis, content analysis, and accurately predict changes in rankings. Some suitable algorithms for this purpose include:
- Random Forest: Random Forest is a powerful algorithm that can handle large amounts of data and make predictions based on many different variables. In the context of SERP analysis, Random Forest could be used to analyse competitor keyword rankings and predict changes in these rankings over time.
- Gradient Boosting: Gradient Boosting is another algorithm that can be used for competitor analysis in the SERPs. It works by combining multiple weak prediction models to make a single, strong prediction. In the context of SERP analysis, Gradient Boosting could be used to analyse competitor content and predict changes in rankings based on this analysis.
- Neural Networks: Neural networks can be used to analyse patterns and trends in competitor behaviour, and predict changes in rankings based on these patterns and trends.
Backlink Analysis with machine learning
Backlinks are an important factor in determining the ranking of a website on the SERP. Machine learning algorithms can be used to analyze the quality of a website’s backlinks and identify any areas for improvement. This information can then be used to optimise the backlink profile of a website and improve its ranking on the SERP.
One algorithm that can be used for backlink analysis is classification analysis. This type of machine learning algorithm can be used to categorise backlinks as either high-quality or low-quality and provide insights into how the quality of backlinks is affecting a website’s ranking on the SERP.
Another algorithm that can be used for backlink analysis is clustering analysis. Cluster analysis is a method of data analysis that uncovers the inherent groupings or clusters present in a dataset so in digital marketing this technique can be used to group similar backlinks together and provide insights into how backlinks are impacting the ranking of specific keywords.
Content Analysis with machine learning
Machine learning algorithms can be used to analyse the content on a website and provide insights into its performance. By analysing large amounts of data, machine learning algorithms can identify the types of content that are performing well and those that are underperforming. This information can then be used to optimise the underperforming content of a website and improve its ranking in the SERPs.
One algorithm that can be used for content analysis is Natural Language Processing (NLP). This type of machine learning algorithm can be used to analyze the content on a website and provide insights into its performance. For example, NLP algorithms can be used to identify the topics covered in a website’s content and how well these topics are resonating with the target audience.
Another algorithm that can be used for content analysis is sentiment analysis. This type of algorithm can be used to determine the tone of a website’s content and identify any areas for improvement.
Classify SEO keywords using GPT-3
We can not talk about content analysis with machine learning without talking about GPT-3 (Generative Pre-trained Transformer 3) – a language model relying on deep learning that can generate human-like texts based on a given text-based input – which has truly revolutionised the field of natural language processing. One of the key applications of GPT-3 is the ability to classify keyword context. This has numerous practical applications, such as sentiment analysis, topic modelling, and content classification to mention a few.
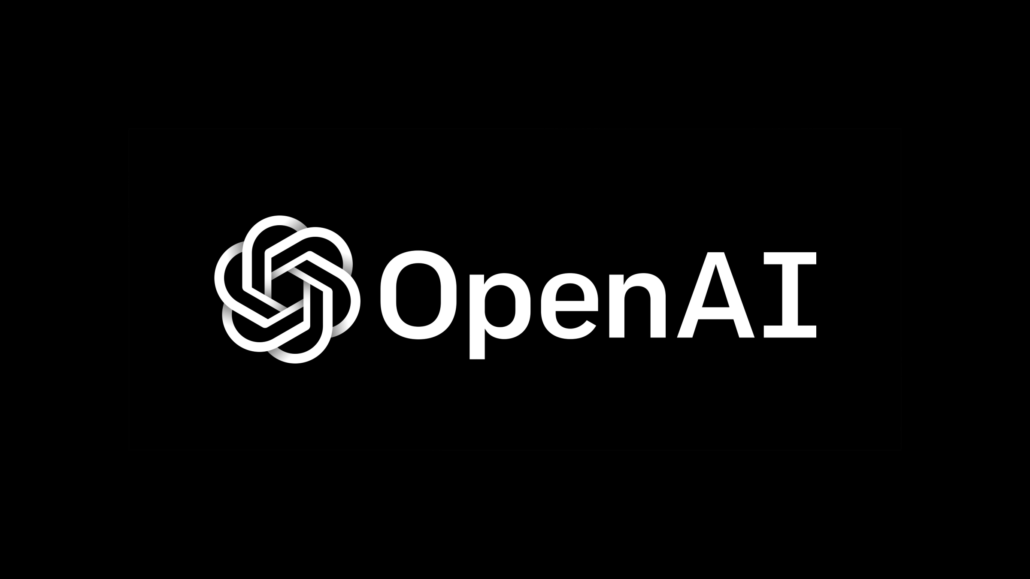
The simplest way to explain the method of classifying the context of specific keywords: GPT-3 is fed with a set of keywords utilising its powerful language processing capabilities to determine the context in which these keywords are used. Before that, the pre-trained GPT-3 model has to be fine-tuned on a large corpus of text data (a large and structured collection of text data) that includes the target keywords and their surrounding context. This pre-training of GPT-3 allows the model to learn the contextual relationships between the keywords and the surrounding text, resulting in GPT-3 accurately classifying new keywords’ contextual relationships in real time.
By far one of the biggest advantages of using GPT-3 for keyword context classification is its ability to handle complex, nuanced language. Unlike traditional keyword-based approaches, which may struggle to understand the context in which keywords are used, GPT-3 has a deep understanding of language and can accurately classify context even in the presence of subtle linguistic cues. This renders it particularly useful for applications such as sentiment analysis, where the context of keywords is critical to accurately determine the overall sentiment expressed in a piece of text.
Last but not least GPT-3 is the perfect machine learning model for keyword context classification because of its ability to handle large amounts of data. The pre-trained model has been trained on a massive corpus of text data, giving it the ability to handle large amounts of text data quickly and accurately. This makes it particularly useful for applications such as topic modelling, where large amounts of text data must be processed to accurately classify topics.
Meet Danny Richman the GPT-3 SEO guru
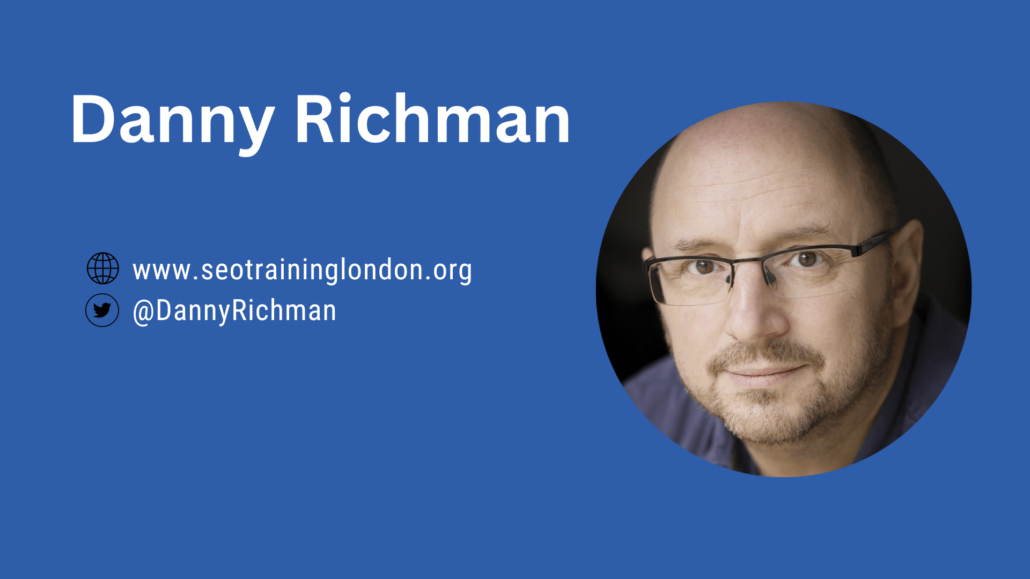
Award-winning SEO expert Danny Richman is a well-known expert in the field of natural language processing and application using GPT-3. Danny Richman has been at the forefront of exploring its potential for various applications, including keyword context classification, advanced sentiment analysis and topic modelling algorithms.
Richman’s expertise and contributions to the field have made him a respected thought leader and a go-to resource for companies looking to leverage GPT-3 for keyword context classification and other natural language processing tasks in digital marketing and SEO.
I strongly recommend that you go through Danny Richman’s Free GPT-3 Sheets for Marketers
Machine learning can boost your predictive analytics
Believe it or not, machine learning algorithms can be used to predict future trends and patterns in the search engine results. By analysing large amounts of data, machine learning algorithms can identify patterns in the ranking of specific keywords and provide predictions on how these rankings may change in the future. This information can then be used to make data-driven decisions about the optimisation of a website and improve its ranking in the SERPs.
One common machine learning algorithm used for predictive analysis is time series analysis. Without going into details time series analysis is a method of analysing a series of data points collected over a period of time. With that in mind, this technique can be used to identify patterns in the ranking of important keywords over time and provide predictions on how these rankings may change in the future.
Another algorithm that can be used for predictive analysis is neural networks. This type of algorithm can be used to make predictions based on historical data and provide insights into the factors affecting keyword rankings.
Note: It’s important to note that these are just a few examples of the many machine learning algorithms that can be used for SERP analysis. The specific algorithm used will depend on the type of analysis being performed and the data available.
It’s also important to work with an experienced data scientist or machine learning expert to ensure that the algorithm used is appropriate for the analysis being performed and that the results are accurate and valuable.
Benefits of using machine learning in SEO
Machine learning is rapidly transforming the way businesses approach search engine optimisation as there are many benefits to integrating machine learning into SERP analysis for SEO purposes. Without a doubt, machine learning algorithms can provide more accurate and valuable insights into the performance of a website in the SERPs.
By analysing enormous amounts of data, machine learning algorithms can recognise patterns and trends that would not be possible to identify through manual ‘human’ analysis. This information can then be used to make data-informed decisions about the optimisation of a website and improve its ranking in the SERPs.
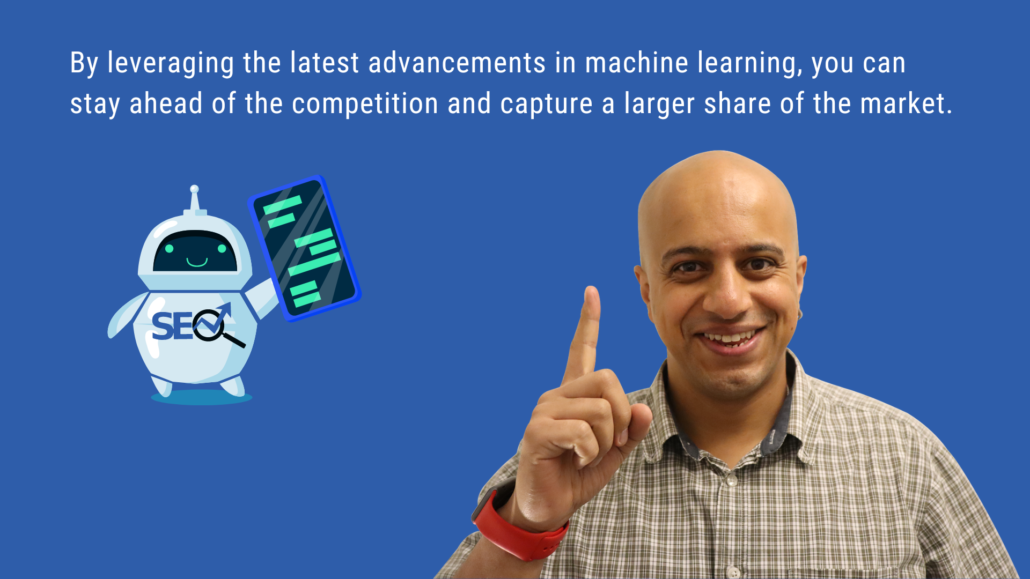
Another benefit of incorporating machine learning into SEO is the ability to automate many of the manual processes involved in SERP analysis. Machine learning algorithms can be programmed to analyse large amounts of data and provide insights into the performance of a website in the SERPs without human intervention. This can save companies time, money and resources, allowing them to focus on other aspects of their digital marketing strategy.
In conclusion, incorporating machine learning into your SERP analysis can provide your businesses with the opportunity to gain more accurate and valuable insights into the performance of your online properties in the SERPs.
With the ability to automate many of the manual processes involved in SERP analysis, machine learning algorithms can help your businesses save time, money and resources while improving the overall performance of your website in the SERPs. By leveraging advanced data analysis techniques, machine learning can provide a more comprehensive understanding of user behaviour and help online businesses improve their rankings, user engagement, and bottom line.
Who is Lazarina Stoy?
Lazarina Stoy is a multi-talented professional who excels in various areas, including being a Technical SEO Analyst, a freelance SEO Growth Strategist, and a gifted storyteller. Her technical prowess and data-focused approach have earned her the title of a progress-driven automation enthusiast.
With a strong desire for enhancement, Lazarina is an avid supporter of the use of automation and machine learning in SEO practices. She has a talent for spotting improvement opportunities in all that she does, making her a valuable contributor to the field of search engine optimisation.
Leave a Reply